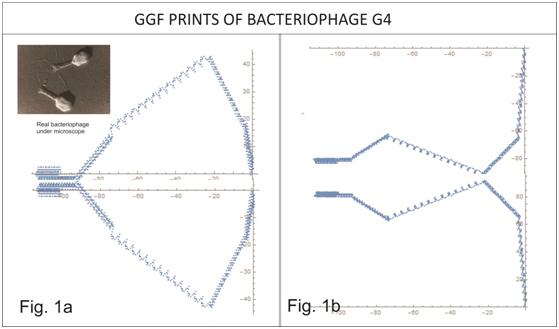
GGF scientific hypothesis
The discovery of the DNA code of 4 bases coding for 20 amino acids has been followed by further discoveries of the triplet code, genes, splicing, protein synthesis etc. However, beyond matching triplet base codes to each of the 20 amino acids and genes to molecular structure or morphologies, just precisely how the DNA code works in a mathematical sense has eluded researchers. Medical scientist and surgeon Dr Brian E. Hagan (1928 – 2007) made a discovery which could be of considerable significance in decoding DNA and the process by which genes express proteins, the configuration of protein molecules and what morphology becomes the body plan of the organism. He developed the algorithm which we now call the Generative Genome Function (GGF) which he applied to the G4 Bacteriophage. Although his GGF algorithm was ‘blind’ as to the G4 body plan morphology ie it was not loaded with any images , morphological or positional information of the G4 body plan (other than the 5577 bases of the G4 genome itself) the algorithm ‘drew’ the morphology of the G4 phage. The GGF algorithm via a number of functions mapped the G4 DNA 5577 bases to a list of results and these results were then entered on a 2 D Cartesian Graph to produce a diagram (see Figs. 1a & 1b above). We call this a “GGF Print” or GGF motif. The algorithm is now enshrined in a GGF software suite (GGF Geneseeker) suitable for researchers or labs to use which can generate up to 15 images at a time including 3D modelling. The GGF takes DNA code and accesses the DNA code mechanism to produce apparent morphology of a microbe, chamber walls of a human mitochondrion, the wing or legs of the Drosophila Melanogaster fly and distinct GGF motifs from the 16S RNA chain in the ribosome. The fact that GGF accesses the 16S RNA chain in the ribosome indicates the GGF may access an ancient mechanism and as such there is a good chance that it is accessing a mechanism that is ubiquitous in all DNA – it may be more than morphology ie it may be a coding mechanism itself with the GGF generated images mapping mere outcomes of a greater general mechanism. Analysis to date indicates that GGF motifs or shorter sequences may portray generic sub units of protein molecules whereas longer sequences may portray 2D profiles of morphologies of all or part of the organism encoded by the DNA processed. Thus, we need a ‘Rosetta Stone’ in biochemical terms between the GGF DNA mapping to amino acids through to the polypeptide chain through to the folding or in the linking together of polypeptides (ie proteins). This Rosetta Stone will probably look like a table and will probably involve mathematical principles governed by rules. However we are already designing a neural network to tune extrapolation of peptide sequences and DNA encoding those sequences using a set of inverse GGF algorithms as part of our deep learning (AI) strategy.